Information Theory and Estimation Theory
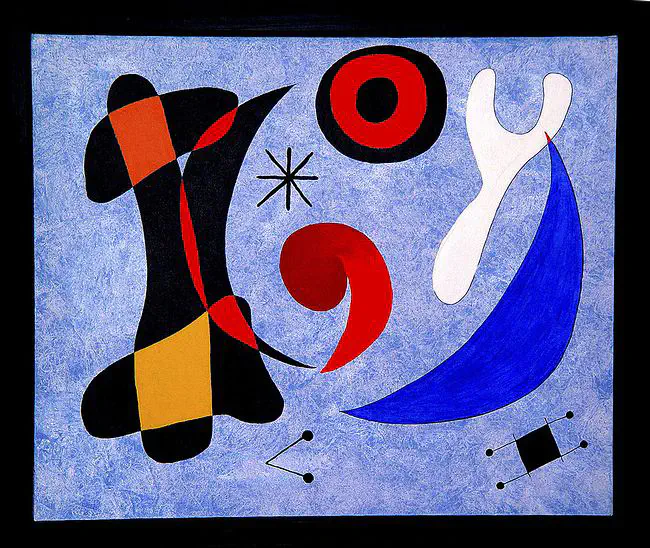
Information theory and estimation theory have generally been regarded as two separate theories with little overlap. Recently, however, it has been recognized that the relations between the two theories are fundamental (e.g., relating the mutual information with the minimum mean-square error) and can indeed be very useful to transfer results from one area to the other. In addition to the intrinsic theoretical interest of such relations, they have already found several applications such as the mercury/waterfilling optimal power allocation over a set of parallel Gaussian channels, a simple proof for the entropy power inequality, a simple proof of the monotonicity of the non-Gaussianness of independent random variables, and the study of extrinsic information of good codes.
We have further explored these connections in the vector Gaussian and arbitrary (non-Gaussian) settings. One interesting application of such a characterization is the efficient computation of the mutual information achieved by a given code over a channel via the symbolwise a posteriori probabilities (which previously could not be computed). We have also considered an alternative definition of information termed lautum information (different from mutual information).
Papers
- Ronit Bustin, Miquel Payaró, Daniel P. Palomar, and Shlomo Shamai, “On MMSE Crossing Properties and Implications in Parallel Vector Gaussian Channels,” IEEE Trans. on Information Theory, vol. 59, no. 2, pp. 818-844, Feb. 2013.
- Eduard Calvo, Daniel P. Palomar, Javier R. Fonollosa, and Josep Vidal, “On the Computation of the Capacity Region of the Discrete MAC,” IEEE Trans. on Communications, vol. 58, no. 12, pp. 3512-3525, Dec. 2010.
- Miquel Payaró and Daniel P. Palomar, “Hessian and Concavity of Mutual Information, Differential Entropy, and Entropy Power in Linear Vector Gaussian Channels,” IEEE Trans. on Information Theory, vol. 55, no. 8, pp. 3613-3628, Aug. 2009.
- Daniel P. Palomar and Sergio Verdú, “Lautum Information,” IEEE Trans. on Information Theory, vol. 54, no. 3, pp. 964-975, March 2008.
- Daniel P. Palomar and Sergio Verdú, “Representation of Mutual Information via Input Estimates,” IEEE Trans. on Information Theory, vol. 53, no. 2, pp. 453-470, Feb. 2007.
- Daniel P. Palomar and Sergio Verdú, “Gradient of Mutual Information
in Linear Vector Gaussian
Channels,” IEEE
Trans. on Information Theory, vol. 52, no. 1, pp. 141-154,
Jan. 2006.
📈 Highly cited paper (ISI Web of Knowledge)